AZoRobotics speaks with Grzegorz Sochacki from the University of Cambridge about his research into the creation of the revolutionary robot chef. The findings of the research could lead to the improvement of semi-automated or automated meal preparation by helping robots learn what tastes good and what does not, thus enhancing their cooking abilities.
In a health-conscious society, diet has become an ever-increasingly important aspect of everyday life. What inspired your research into fabricating a robot chef that is able to analyze the saltiness of food?
Salt is the most common seasoning and is also present in raw ingredients; therefore, when implementing this sense, we can assess both the seasoning state and ingredient ratios. Therefore, in one fast measurement, we get a sensing stage for both cooking and seasoning control loops.
Moreover, the salinity is measured by measurement of conductance, capturing information about other food properties by proxy. For example, extra humidity improves contact between electrodes and food and what can be picked up by the sensor.
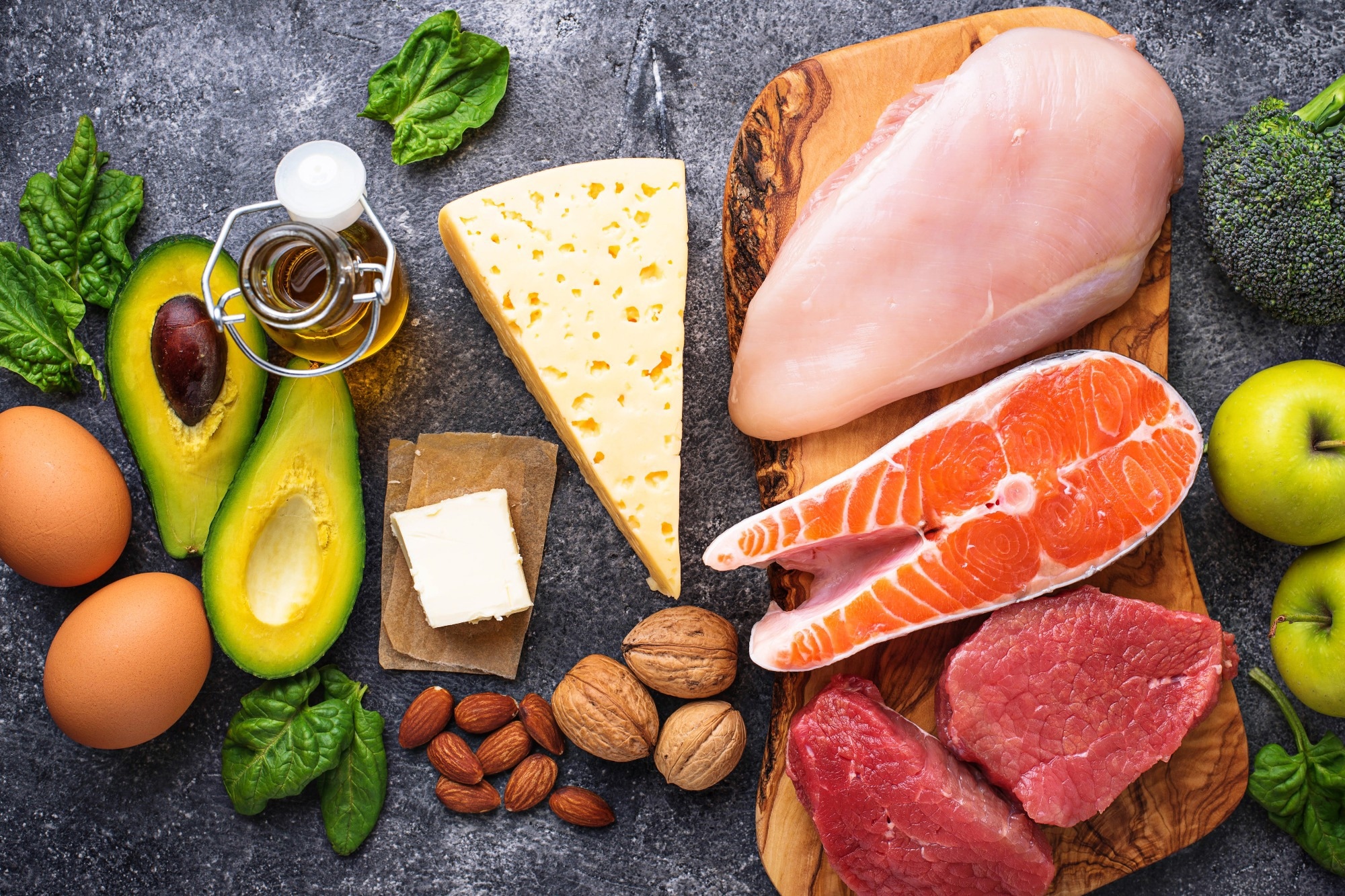
Image Credit: Shutterstock.com/Yulia Furman
Culinary arts is one of the activities where humans dominate over automated robotic systems. What are some of the challenges that have previously hindered the implementation of artificial intelligence in the culinary process?
Currently, the most impressive results in machine learning are achieved using deep learning. The algorithms are extremely data hungry and therefore usually used only in fields where data is cheap, e.g., images or text. Collecting relevant datasets for cooking is much harder, troublesome and more expensive.
Firstly, preparing a dish is expensive. Secondly, tasting it by humans takes time, is subjective, and hard to compare, as even a single human taster will have a hard time remembering some of the dishes they tasted a while back.
Therefore, we believe that robot chefs, by lowering the cost of large-scale experiments, can solve these problems and enable machine learning in the field of taste sensing and robotic cooking.
Moreover, sensing is harder than in most applications, mainly due to the need for contact sensing of quite complicated substances that are used in most dishes.
Automation of culinary tasks requires many of the abovementioned challenges to be addressed. How did your team overcome the challenges traditionally associated with automation in the culinary field?
Automation in-home environment is always challenging, especially with limited time and resources. We find it very useful to start with the as simple as possible setup and then add only the complexity that is necessary. This makes a minimal setup that can be adjusted quickly while the project is evolving. Moreover, we have found out that mounting the robot on a mobile platform gives a lot of flexibility.
Another interesting part is using kitchen utensils with rubber elements – they are stiff enough for most tasks yet give some slack for position control. Having these soft elements helps when two metal elements come together – for example, when scraping eggs from the bottom of a pan, they introduce an automatic force control.
On the other hand, in the case of sense, we have noticed how big of an effect mastication has on tasting. We have found out that different dishes react differently to mechanical processing, and it can be used to make classification tasks easier. Moreover, we now understand how important the water content is for sensors (and taste buds) to work properly. A large number of these sensors are sensitive to ion concentration.
Therefore, the role of saliva is crucial for tasting. Extra humidity and crushing of the food help with contacting the sensor to the food and influencing measurements greatly. Incorporating mastication effectively changes the challenges of food sensing into an extra source of information.
Can you provide an overview of how your robot chef is able to replicate the process of tasting and adjusting the flavor of food?
Assessing food palatability or tasting is based on measuring a few basic tastes like sweetness, sourness, and saltines, as well as a few more features like temperature, humidity or smell. We believe that capturing enough of these signals can implement sensing that is indistinguishable from human taste.
In our studies, we started with conductance measurements taken at various points of the dish. We then computed the mean and variance of the samples. These metrics describe overall saltiness and mixedness.
The robot can “taste” these metrics in an example dish cooked by a human, collecting a benchmark for a well-cooked dish. The robot can then attempt to cook its dish so that it tastes the same.
This brings us to a point where the robot knows the goal value of these metrics and their value for its dish. At this moment, these numbers can be fed into a control loop, changing parameters like the time of pressing a salt mill and the amount of mixing.
Taste of the future: robot chef learns to ‘taste as you go’
Taste of the future: robot chef learns to ‘taste as you go’ Video Credit: University of Cambridge
When it comes to tasting food, there are many parameters that must be considered, such as sweetness, saltiness, and tartness. Why did you opt to measure salinity levels in your preliminary studies?
While we know sensors that can measure other basic tastes, the variety of available food types and their consistency, such as stickiness, prompts the use of sensors that are easy to clean and that are least dependent on the consistency and contact area with the food.
Theoretically, these limitations could be bypassed by pre-processing the samples and sampling it in the laboratory environment, but this is not only destroying most of the information but also is not suitable for use in kitchen use.
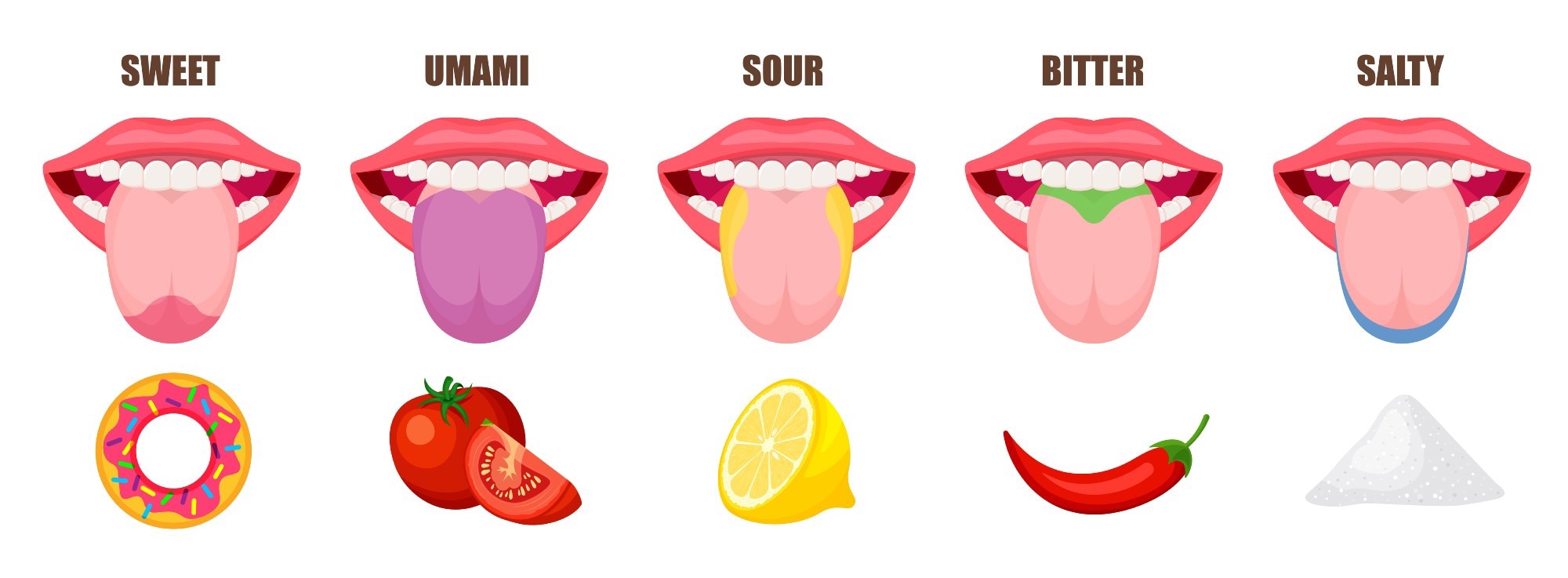
Image Credit: Shutterstock.com/Yuliia Konakhovska
Therefore, our mission was to find the most simple method of implementation of sensing possible. That is the thought chain bringing us to a conductance sensor as it can measure solids (unlike ion-selective sensors that require water solution to work), is easy to clean between measurements (two platinum rods to be scraped), and is easy to ensure good contact between the sensor and the sample (sensor pierces the food).
Can you explain how you set up the robotic salinity tests and what were the results of such experiments?
The birdseye view of our setup is a robotic arm with a salinity probe attached to it. Various probes are available, as well as robotic arms. For the probe, we went with a conductance sensor, which is made out of two platinum electrodes.
AC voltage is set across these with a control circuit, and the resulting current is measured. It may be thought of as a measurement of how hard it is to push current between the electrodes. This value is dependent on factors of the sampled food itself, seasoning (amount of salt), humidity, and texture (more air gaps are more resistant to current).
Taking numerous samples, we can make an “image” or “map” of the taste that can be used for machine learning. If we add chewing on top of that, we get a video of how taste is changing along with mechanical processing.
Are there any challenges or limitations of the robot chef? How does it compare to using humans in the evaluation of food?
Our research on robotic chefs is in a very early stage, and we seek to match human performance. Such a feat will require the use of smell and vision on top of taste, as well as a lot of data.
We must also note that a lot of knowledge and common sense are used to evaluate food. For example, cold scrambled eggs are undesirable, while it is the opposite for beer. It follows with other features, too; for example, we expect lemon to be sour, but sourness in a vegetable soup usually means it is spoiled. It works similarly with tactile experience, crispy chips are considered tasty, but it would mean old or burned for other foods.
Therefore, we believe that sensing is not enough to replicate the human experience, and we need something close to perception. We need to implement the robotic chef with the ability to sense and weigh the outputs against its prior knowledge and expectations.
What are the potential applications of this novel robotic chef?
I look forward to applying the robotic chefs in industry and the home. We have seen the cost of hardware and the cost of adjusting the robot for a specific kitchen as the largest bottlenecks.
Taking these limitations into account, we expect the technology to initially be implemented in highly valued areas like elderly care or in more structured environments like kitchens in franchise restaurants.
The first one is a case where the robot’s work is valued much higher than the produced meal itself, as it provides independence for the elderly. In the other case, the robots reduce costs in large kitchens and can operate for a significantly longer period of time, spreading the costs even further.
What industries would benefit from this novel technology? Who is your main target customer?
I acknowledge that the overheads required to implement a robotic chef in a restaurant are quite big. Therefore, we aim for places that can make the most of them. Therefore, we aim for places that operate 24/7 or in high labor cost areas. Perhaps airport restaurants could be a good place for the first implementation. Dark kitchens could also be a viable application area. I also hope to dive deeper into commercialization in around a year after I have completed my Ph.D.
After the success of your initial study, what are the next steps for your research?
The next step is to implement more senses into robotic cooking. Sourness is a good candidate to do this as it is one of the basic tastes that can be replicated with an existing sensor. Moreover, both smell and visual clues have a huge role in human taste and one’s enjoyment of food.
Therefore we believe a complete robotic chef should use them. We also look at how these senses could be used for cooking with less structure to the environment, e.g., Introducing a significant variability to the food produced.
When it comes to commercializing the robot chef, what equipment would you need, who would provide it, and what partners do you hope to accompany you on this journey?
I believe that the best way to commercialize the robot chef is to use as many off-the-shelf components as possible while leveraging the sensor and perception technology we developed. This is mainly due to the fact that a lot of capabilities can be bought for a reasonable price and have proven reliability. Moreover, the robotic arms should also be used sparingly. They are great for fast prototyping due to their flexibility, but they may be overkill in many purpose-built setups. In the case of commercialization, a spin-out company would probably work best.
About Grzegorz Sochacki
Grzegorz Sochacki is a Ph.D. student at the University of Cambridge in a Bio-Inspired Robotics Laboratory, working on robotic taste and the automation of high-margin meal cooking.
Disclaimer: The views expressed here are those of the interviewee and do not necessarily represent the views of AZoM.com Limited (T/A) AZoNetwork, the owner and operator of this website. This disclaimer forms part of the Terms and Conditions of use of this website.