Artificial intelligence has been driven by advances in computational technology, resulting in an expanded ability to collect and process large volumes of data, which have been essential in drug discovery.
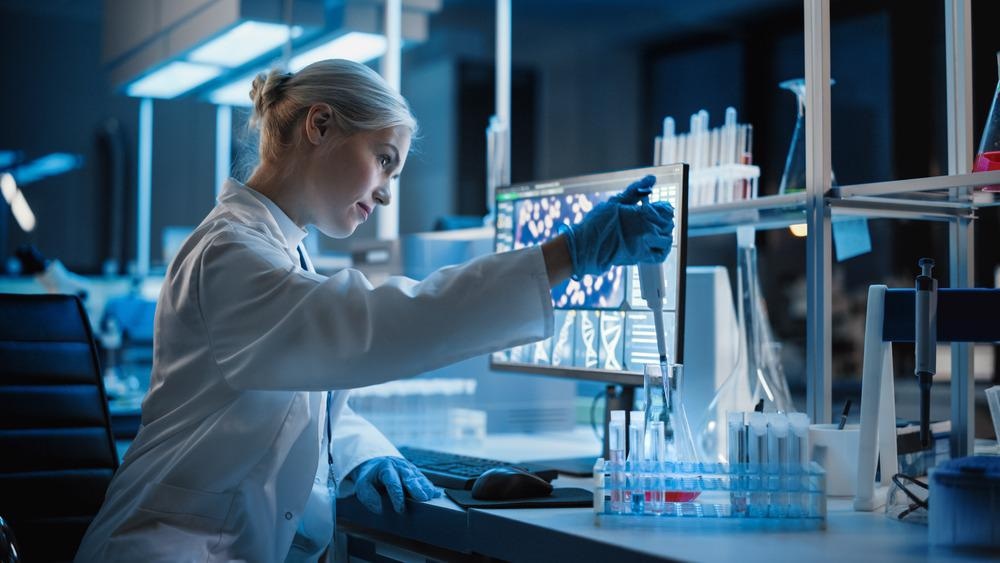
Image Credit: Gorodenkoff/Shutterstock.com
Alongside this, costs of bringing new drugs to market have increased; the development of a new drug is a long and expensive process with a low success rate.
Given these limitations, artificial intelligence techniques, due to their automated nature, efficiency, and predictive accuracy, possess the potential to increase the efficiency of drug development. This has, and will continue to, subsequently reduce the cost, decrease development timelines, and increase the likelihood of success.
Artificial intelligence is likely to change the way that clinical trials are designed, operated, and analyzed. Following the global SARS-CoV-2 (COVID-19) pandemic, the use of artificial intelligence in the research, science, and processes required for drug development (R&D) will undoubtedly accelerate. Furthermore, the pandemic has increased dependency on digital technology for data collection – and artificial intelligence has occupied a greater role in the process, expanding its possibilities.
What is Artificial Intelligence?
Artificial intelligence is a tool capable of creating systems that mimic human behavior through the use of algorithms. Machine learning achieves artificial intelligence by using algorithms that have been trained with a wide range of data. Following this, deep learning can be achieved; these systems are analogous to the brain's structure and produce artificial neural networks.
Artificial intelligence techniques increase the likelihood of successful drug development by improving different aspects of R&D. Areas include identifying novel targets, selecting viable drug candidates, designing and optimizing molecular compounds and developing prognostic and predictive biomarkers.
The Clinical Design Process
In the context of preclinical research, artificial intelligence is being used by way of natural language processing to glean scientific insights from biomedical literature, insurance claims, and medical records to identify novel targets. In addition, predictive modeling can be used to forecast protein structures and help with molecular compound design and optimization to produce a suite of drug candidates that are more likely to be effective.
In the postmarketing state of drug production and development, artificial intelligence is predicted to play a role in the mining of big data from real-world sources to increase understanding of the benefit-risk profile of a drug, understand treatments, and identify a subcategory of patients who may be particularly amenable to the effects of treatment. Some of these applications are already underway.
Examples of Artificial Intelligence in Action
Several instances demonstrate the use of artificial intelligence methods being used in drug discovery.
Protein Structure Prediction Using Deep Learning for Drug Repurposing
Artificial intelligence has been used for protein structure prediction and drug re-purposing. Generally, the biological mechanism of a protein can be inferred from its three-dimensional structure, which is encoded by its one-dimensional string of amino acids. Protein structure can help discover new therapies that are not aimed at inhibiting or activating specific target proteins.
Within the protein folding domain, protein misfolding is known to be a pathological determinant of several diseases, including Alzheimer's, Huntington's, Parkinson's, and type 2 diabetes. There is still a knowledge gap between amino acid sequence and determination of three-dimensional structure; therefore, artificial intelligence has been used to accurately predict 3D protein structures to better understand the nature of protein folding disease and facilitate the development of new drugs.
An example comes from the program AlphaFold, developed by DeepMind; this is an artificial intelligence network used to determine the shape of a protein-based on its amino acid sequence. The program was trained on the protein data bank structures, predicting distances between pairs of amino acids to give a probabilistic estimate in a defined region of a distance map.
All of these regions are tiled together to produce the distance predictions across the entire protein that fulfills amino acid distance rules. Taking it further, a research team developed a deep learning-based drug-target interaction prediction model that predicted how well the chemical sequences in a drug bound to the amino acid sequences of a protein target without structural information.
The team successfully identified several existing antiviral drugs which demonstrated uninhibited tree ability against the SARS-CoV-2-like proteinase, demonstrating the potential re-purposing of these antiviral drugs as candidate treatments for SARS-CoV-2.
In 2020, structure predictions of five understudied SARS-CoV-2 variants were released by AlphaFold.
Translation of Research and Precision Medicine: Developing Predictive Biomarkers
Artificial intelligence by way of machine learning has been used across several published research to stratify patients in clinical development. For example, predictive models were produced to query whether the models that were based on the cell line screen data could predict how well a patient responded to treatment for non-small cell lung cancer and pancreatic cancer (erlotinib) and a treatment for kidney, liver, and thyroid cancer (sorafenib).
In a further study, the predictive model stratified patients who were receiving erlotonib in a clinical trial. The model-predicted erlotinib-sensitive patient group demonstrated a median progression-free survival (PFS) or 3.84 months, while those patients predicted to be resistant demonstrated a PFS of 1.84 months.
This suggested that the erlotonib-sensitive patients had a more than double benefit to PFS relative to resistant patients. A similar effect was seen in patients receiving sorafenib; the model predicted sorafenib-sensitive patients had a PFS of 4.53 and the model predicted sorafenib-resistant patients had a PFS of 1.87.
MIT Quest for Intelligence Launch: AI-Driven Drug Discovery
MIT Quest for Intelligence Launch: AI-Driven Drug Discovery. Video Credit: Massachusetts Institute of Technology (MIT)/YouTube.com
Non-Parametric Bayesian Learning for Clinical Trial Design and Analysis
Non-parametric Bayesian learning is a tool that has emerged in the artificial intelligence space. It enables a non-parametric approach to selecting models. Normally, common machine learning methods are focused on learning several parameters within models, called model selection. But this results in overfitting or underfitting of the model.
This method is applied in clinical trial design. For example, non-parametric Bayesian learning can enable efficient and effective selection of doses for patients. This overcomes a common problem in oncology trials; patients are often heterogeneous, presenting with multiple types of cancer, which can be a pronounced issue for those receiving Immuno-oncology and cell therapy.
This is because heterogeneity can cause differences in safety or efficacy profiles, resulting in an imprecise dose selection and an inability to identify stratified patient populations who will respond most efficiently.
Researchers have successfully employed the method of non-parametric Bayesian learning to improve the dose search efficiency and the optimal dose level accuracy of the estimates proposed for a stratified group.
Current Position of Artificial Intelligence in Clinical Trial Design, Limitations, and Future Direction
Although artificial intelligence is currently widely used in drug discovery and translational research, its role in clinical trial operations has been less successful.
This is because of the challenges faced by patient recruitment ~ 80% of trials do not meet their enrollment timelines; in addition, ~ 30% of phase three clinical trials are terminated early due to enrollment. Generally, the monitoring of clinical trials is labor-intensive, time-consuming, and expensive.
However, artificial intelligence could play an important role at the point at which the last phase three trial is conducted to submit the data package for regulatory approval. AI can shorten the duration at this point, which will expedite the rate at which drugs are given to patients and reduce costs.
Artificial intelligence is also predicted to play an important role in cleaning and locking trial data, producing analysis results which include data listings, figures, and summary tables, producing the final clinical study report, integrating safety and efficacy data, and creating data submission packages.
As a result of the COVID-19 pandemic, "decentralized" trials, in which patient participation can be assessed and included virtually, will enable the greater capability for artificial intelligence to collect and analyze patient data.
Moreover, artificial intelligence and machine learning technologies are hoped to augment the success of patient recruitment and project enrolment, as well as allow for real-time automated and smart monitoring of data quality, as well as effective monitoring of clinical trial site performance.
References and Further Reading
Kolluri S, Lin J, Liu R, Zhang Y, Zhang W. Machine Learning and Artificial Intelligence in Pharmaceutical Research and Development: a Review. AAPS J. 20224;24(1):19.https://doi.org/10.1208/s12248-021-00644-3
Senior A, Evans R, Jumper J, et al. Protein structure prediction using multiple deep neural networks in the 13th Critical Assessment of Protein Structure Prediction (CASP13). Proteins. 2019;87:1141–8. https://doi.org/10.1002/prot.25834
Senior A, Evans R, Jumper J, et al. Improved protein structure prediction using potentials from deep learning. Nature. 2020;577.https://doi.org/10.1038/s41586-019-1923-7
Beck BR, Shin B, Choi Y, et al. Predicting commercially available antiviral drugs that may act on the novel coronavirus (SARS-CoV-2) through a drug-target interaction deep learning model. Comput Struct Biotechnol J. 2020;18:784–90. https://doi.org/10.1016/j.csbj.2020.03.025
Li B, Shin H, Gulbekyan G, et al. Development of a drug-response modeling framework to identify cell line-derived translational biomarkers that can predict treatment outcome to erlotinib or sorafenib. PLoS ONE. 2015;10(6):e0130700. https://doi.org/10.1371/journal.pone.0130700
Li M, Liu R, Lin J, et al. Bayesian semi-parametric design (BSD) for adaptive dose-finding with multiple strata. J Biopharm Stat. 2020;30(5):806–20. https://doi.org/10.1080/10543406.2020.1730870
Disclaimer: The views expressed here are those of the author expressed in their private capacity and do not necessarily represent the views of AZoM.com Limited T/A AZoNetwork the owner and operator of this website. This disclaimer forms part of the Terms and conditions of use of this website.